Machine Learning for Drug Discovery (MEAP V01)
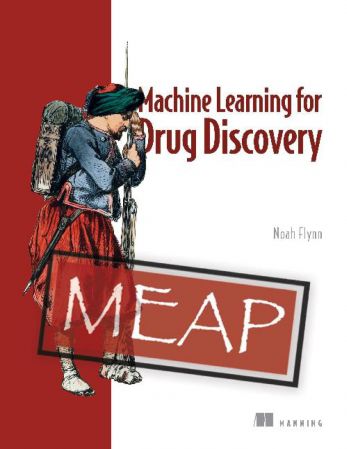
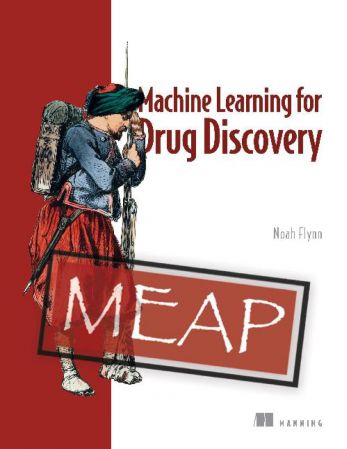
Machine Learning for Drug Discovery (MEAP V01)
English | 2024 | ISBN: 9781633437661 | 245 pages | PDF,EPUB | 27.7 MB
Discover how machine learning, deep learning, and generative AI have transformed the pharmaceutical pipeline as you get a hands-on introduction to building models with PyTorch.
Machine Learning for Drug Discovery introduces the machine learning and deep learning techniques that drive modern medical research. Each chapter explores a real-world example from the pharmaceutical industry, showing you hands-on how researchers investigate treatments for cancer, malaria, autoimmune diseases, and more.
In Machine Learning for Drug Discovery you will learn
Drug discovery and virtual screening
Classic ML, deep learning, and LLMs for drug discovery
UsingRDKit to analyze molecular data
Creating drug discovery models with PyTorch
Replicating cutting-edge drug development research
Machine learning has accelerated the process of drug discovery, shortening the timeline for developing new medicines from decades to years or months. In this practical guide, you’ll learn to create the kind of machine learning models that make these discoveries possible.
about the book
Machine Learning for Drug Discovery introduces the fundamentals of drug discovery and cheminformatics along with the machine learning techniques used by leaders in the pharmaceutical industry. Each chapter guides you through an engaging hands-on project that explores a real medical issue. You’ll build a full screening pipeline to assess a compound’s potential for treating malaria, reproduce published methods for HIV drug design, learn to use deep generative models for novel drug optimization, and see how LLMs can overcome common problems of protein folding
about the reader
All you need are the basics of Python. This book will teach you everything else.
about the author
Noah Flynn is a research scientist at Amazon with a PhD in Computational Biology from Washington University in St. Louis. He has developed deep learning applications to screen drugs for bioactivation, reactive metabolite formation, drug-drug interactions, and other types of toxicity problems. He has worked at AbbVie and Merckon analysis of gene regulatory networks and protein-protein interactions and applications of generative models to construct and optimize novel compound libraries. He now researches applications of large language models at Amazon.